In recent years, the field of artificial intelligence has witnessed remarkable advancements, particularly in the domain of large language models. These sophisticated systems like ChatGPT have demonstrated impressive capabilities in natural language processing, coding, and problem solving. However, the question remains “how close are we to achieving true artificial general intelligence?”
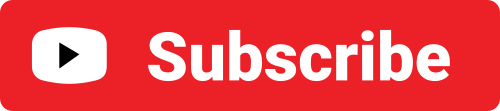
Despite the rapid progress the path to AGI is fraught with challenges and limitations. LLMs, while powerful, still lack crucial components of general intelligence. They struggle with causal reasoning, common sense understanding, and the ability to generalise knowledge across domains. These shortcomings highlight the significant gap between current AI systems and the benchmark for AGI.
The Limitations of LLMs
LLMs such as ChatGPT and Claude have achieved remarkable feats in natural language processing, but they remain far from AGI. These models excel at generating coherent text based on patterns observed in their training data, yet they lack true understanding and reasoning.
As sophisticated as they are, LLMs fundamentally function as advanced statistical pattern recognisers, not as entities capable of abstract thinking or learning from new experiences in real-time. This limitation becomes more pronounced in tasks that require causal understanding or common sense reasoning, areas where AGI would need to excel to match human intelligence.
At their core, LLMs are powerful statistical models trained on vast amounts of text data. They learn to predict the next word in a sentence based on patterns they’ve identified during their training. The process begins with tokenising text into smaller parts, such as words or sub-words, which the model uses to map complex relationships between words and contexts. The model doesn’t “understand” the text in the human sense but can generate highly convincing language based on its exposure to enormous datasets.
- Training and Pattern Recognition
LLMs are trained using supervised learning techniques on billions of words from books, websites, and articles. This helps the model detect patterns in language use, allowing it to generate text, translate languages, or answer questions by predicting the most appropriate sequence of words. - Iteration and Improvement
The model refines its predictions by iteratively generating text. When given a prompt, it identifies patterns it has seen before, which influences its word choices. This process continues until it produces a response that mirrors natural human language. - Limitations in Comprehension and Reasoning
While LLMs can generate sophisticated and contextually appropriate text, they don’t truly comprehend the meaning behind the words. They excel at surface-level language tasks but lack the ability to understand or reason about the real world. Moreover, LLMs struggle with tasks that require common-sense knowledge or learning from new experiences in real-time
Artificial General Intelligence is not simply an advanced pattern-recognition system like current large language models, but rather an entity capable of performing any intellectual task a human can, across various domains. While LLMs are highly specialised in language-based tasks, AGI would need to demonstrate flexibility and adaptability in handling unfamiliar situations, a feat beyond the capabilities of today’s LLMs. LLMs, though impressive, are restricted to generating text based on patterns found in their training data and lack the ability to generalise knowledge across different fields.
Another key difference is that AGI would be able to learn continuously from interactions with the real world, updating its knowledge dynamically. In contrast, LLMs are limited to static, pre-existing datasets. AGI would also need to develop a deeper understanding of cause and effect, enabling it to reason through complex, real-world situations—an area where LLMs currently fall short. This need for reasoning, adaptability, and continuous learning highlights the fundamental gap between current AI models and true AGI
Simply scaling current models or feeding them more data won’t be sufficient to bridge this gap.
Where is ChatGPT5?
Many expected rapid iteration from OpenAI following the release of ChatGPT4, but the development of ChatGPT5 appears to have slowed. Several factors are likely contributing to this delay, including internal challenges at OpenAI.
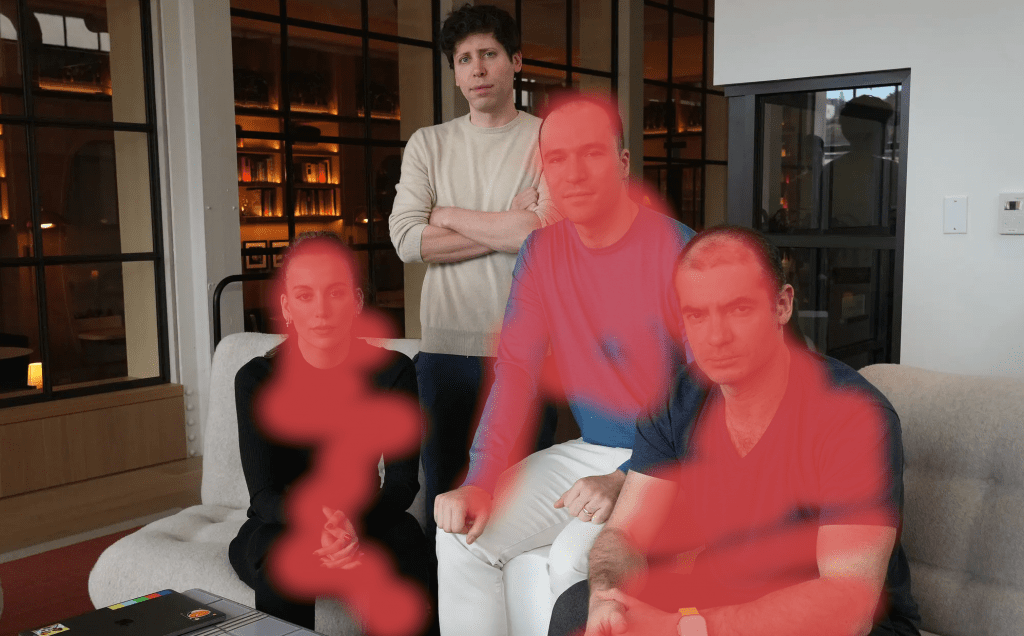
An internal exodus reflects a broader uncertainty within the company about how to manage the ethical and safety challenges posed by the development of AGI.
Additionally, OpenAI’s focus has shifted from purely research driven goals toward product development and commercialisation, which may also be delaying breakthroughs on the path to AGI. Despite significant strides in LLM capabilities, some argue that the challenges of achieving AGI may require entirely new paradigms in AI development.
In the meantime, Anthropic’s Claude 3.5 Sonnet model has rapidly gained ground, becoming a popular choice for text and coding tasks. Claude has shown proficiency in handling complex requests in these domains, offering a credible alternative to OpenAI’s models. However, like ChatGPT, Claude remains an advanced form of narrow AI, adept in specific tasks but limited in scope and understanding.
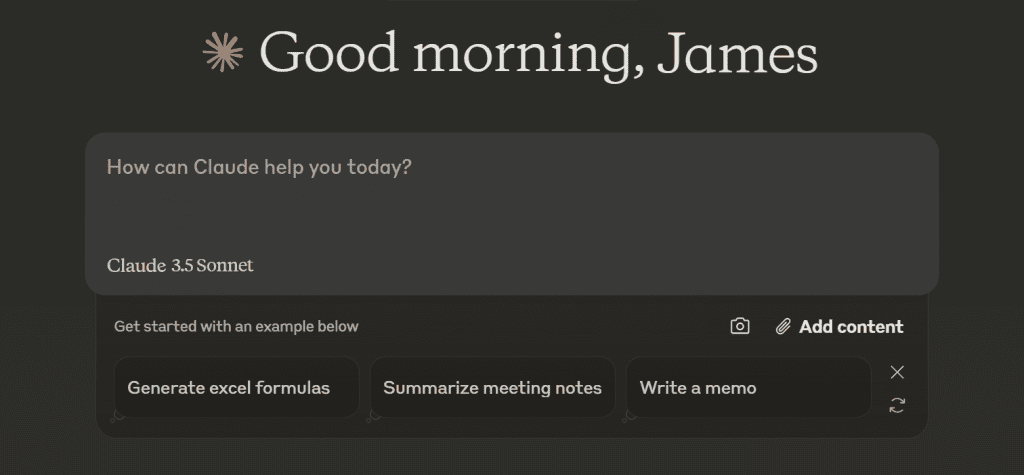
What Defines AGI?
Experts generally agree that AGI would require the ability to perform any intellectual task a human can, including generalisation across a wide variety of fields.
Benchmarks for AGI often include passing the Turing test, exhibiting common-sense reasoning, and demonstrating creativity and emotional intelligence. However, these criteria are subject to debate, with some arguing that true AGI should surpass human capabilities in all cognitive tasks.
For instance, the “Coffee Test” suggests that an AGI should be able to walk into a stranger’s home and make coffee without assistance, demonstrating adaptability in a new environment. Other benchmarks include the ability to write complex code, understand intricate narratives, or convert complex mathematical proofs into formal symbols.
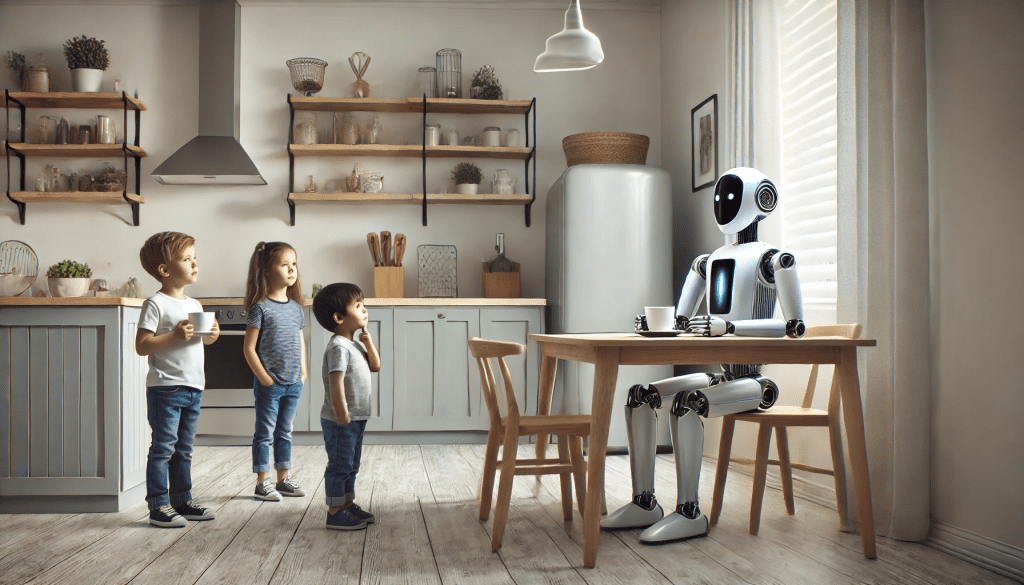
However, passing these benchmarks would only indicate that a system is capable of performing certain tasks, not necessarily that it possesses the reasoning or consciousness akin to human intelligence.
The development of AGI likely requires breakthroughs in understanding how to embed real-world knowledge and causal reasoning into AI systems.
How Far Are We?
Despite the impressive capabilities of current models, AGI remains a seemingly distant goal. Current AI systems are highly specialised and lack the flexibility and adaptability of human intelligence.
Key challenges include building AI that can generalise knowledge across different contexts and domains, and that can handle unexpected scenarios not present in its training data.
In a recent survey by AIMultiple which surveyed 1700 “expert opinions” the mean consensus was that we will achieve AGI around 2040. This is in-line with Sam Altman’s comments in 2024 that it will take “a few thousand days” to get there. Elon Musk suggested we may get “Full AGI by 2029” although he is know for his ambitious timeframes. Shane Legg who is co-founder of Deep Mind stated a “50% chance of AGI by 2028”.
The ethical and safety implications of AGI are becoming more pressing, which has slowed some research efforts as companies grapple with regulatory oversight and how to ensure AGI’s development benefits humanity without creating unforeseen risks.
The consensus is that while AGI may be theoretically achievable within the next few decades, the road there is far from straightforward. Significant technological, ethical, and societal hurdles remain to be addressed before we can consider ourselves close to true artificial general intelligence.